Highly Intelligent Is Not Highly Creative – How to Be Truly Innovative
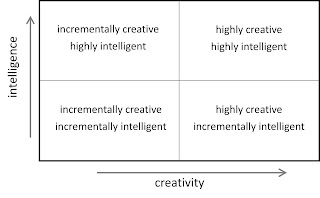
In research as well as in entrepreneurship, there are many characteristics distinguishing an exceptional researcher or entrepreneur from just a good one. In particular there is a key difference between highly intelligent and highly creative people, as these characteristics are not necessarily correlated. Intelligence is commonly defined as the ability to acquire and apply existing knowledge and skills, while creativity is the ability for something novel and valuable. New research and new enterprises can therefore be grouped into four categories: Highly intelligent, incrementally creative researchers or entrepreneurs add an incremental twist to existing methods and knowledge. For instance, a researcher might add mathematical bells and whistles to widely accepted ideas and concepts - in Renaissance Europe they might have added an additional proof that Earth was indeed flat and that the Sun was circling around it. Most of the papers in Nature and Science fall into this category, ce...